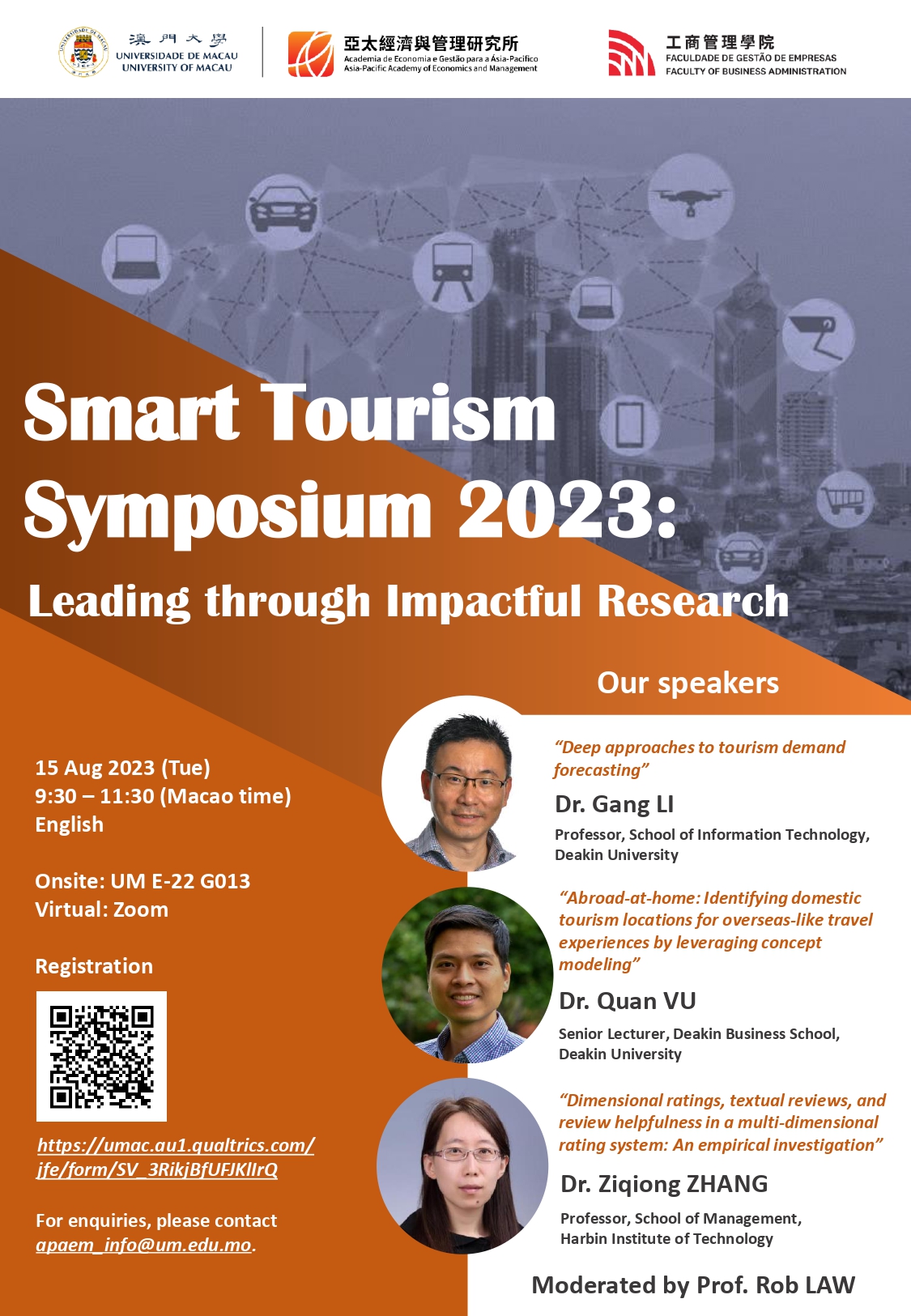
Time | Agenda |
9:30 – 9:40 | Welcome speech by UM representative |
9:40 – 10:05 | “Deep Approaches to Tourism Demand Forecasting” Presented by Dr. Gang LI
Abstract: As tourism researchers continue to search for solutions to determine the best possible forecasting performance, it is important to understand the maximum predictivity achieved by models, as well as how various data characteristics influence the maximum predictivity. Drawing on information theory, the predictivity of tourism demand data is quantitatively evaluated and beneficial for improving the performance of tourism demand forecasting. The methods are available for univariate and multi-variate data. Then we will present a series of deep approaches to tourism demand forecasting – deep learning models, decomposition method, and group pooling method. The empirical results demonstrated that the deep learning approach significantly outperforms alternative models. Moreover, the construction and identification of highly relevant features from the proposed deep network architecture provide practitioners with a means of understanding the relationships between various tourist demand forecasting factors and tourist arrival volumes. |
10:05 – 10:30 | “Abroad-at-Home: Identifying Domestic Tourism Locations for Overseas-Like Travel Experiences by Leveraging Concept Modeling” Presented by Dr. Quan VU
Abstract: Some countries have places with foreign-like scenery that attracts domestic visitors to enjoy overseas-like travel experiences. However, such overseas-like travel experiences have not been widely utilized for domestic tourism development. This is likely due to the lack of awareness among the scientific community and tools for effectively identifying such travel experiences. To address the related gaps and technical challenges, this paper investigates the potential of abroad-at-home as a type of domestic tourism for promoting domestic tourism. This paper also introduces a novel technique called concept modeling that identifies abroad-at-home travel experiences from travel photos taken within a country. A case study on a large-scale dataset of more than 265,000 geotagged travel photos taken in Australia demonstrated the effectiveness of this method. The proposed method and findings are valuable for tourism organizations that use abroad-at-home travel experiences for domestic tourism development. |
10:30 – 10:40 | Break |
10:40 – 11:05 | “Dimensional Ratings, Textual Reviews, and Review Helpfulness in a Multi-Dimensional Rating System: An Empirical Investigation” Presented by Dr. Ziqiong ZHANG
Abstract: Recently, many online review platforms have introduced a multi-dimensional (MD) rating system that provides richer quantitative data to reduce users’ information processing effort and information uncertainty. In an MD rating system, reviewers are required to provide ratings on specific product dimensions, selected by the platform, in addition to providing a textual review. In this paper, we examine how the presence of multidimensional rating affects the provisioning of textual reviews and the consequent effect on textual review helpfulness. Specifically, we leverage four quasi-experiments in a large hotel review website and find that in the review generation stage, the presence of rating on a given dimension can increase the depth of information contained in the textual review about that specific dimension. In the review consumption stage, like the results of past studies, we find that the depth in the textual review of a dimension is positively related to helpfulness. More importantly, we find that such a relationship is moderated by the nature of product dimension. Specifically, we find that when a dimension is listed in dimensional ratings, the positive relationship between the dimension depth and review helpfulness is amplified for a horizontally differentiated dimension but attenuated for a vertically differentiated dimension. |
11:05 – 11:25 | Q&A |
11:25 – 11:30 | Concluding remark |